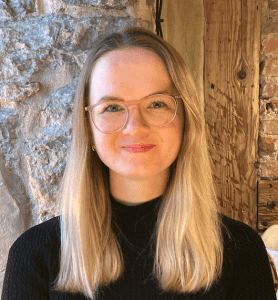
JGI Student Experience Profiles: Marina Vabistsevits (Ask-JGI Data Science Support 2022-23)
What made you decide to apply to join the Ask-JGI team?
I applied to join the Ask-JGI team because I wanted to be involved in a wider data science community at the University of Bristol. I wanted to learn about data-intensive research in other disciplines and possibly gain data science skills outside my PhD project.
What did you find most rewarding about your Ask-JGI experience?
Being a part of the Ask-JGI team meant helping other researchers at the University with their data science queries. The queries we received involved anything from statistics, programming, data visualisation, machine learning, NLP, but also data ethics, data storage, software development, and even public engagement. I enjoyed being exposed to many aspects of data science, which would otherwise be impossible during one PhD programme. The most rewarding part of this experience was researching how to best help the person with their query to find the solution that would work for them, thereby helping them to advance their research at the University.
What sort of work did you do as a part of your Ask-JGI experience?
My favourite queries involved helping people with R programming, often including statistics, data visualisation, and app development. I was also involved in a long-term project with the Professional Services team at the University with another Ask-JGI student. We helped the team with analysing and producing insights from an extensive survey. This allowed the team to make data-informed decisions about the next steps, which may have a University-wide impact.
Another exciting part of my Ask-JGI experience was going to the AI UK 2023 conference in London to present and promote the Data Hazards framework developed by former colleagues at the JGI.
Would you recommend this experience to other students?
I would absolutely recommend joining the Ask-JGI Data Science support team. It is an excellent opportunity to learn about data science outside your immediate field, gain practical knowledge in new areas, and experience a data science consulting role! You also get to meet really interesting people and learn about the broader application of the skills you’re gaining in your current PhD programme.