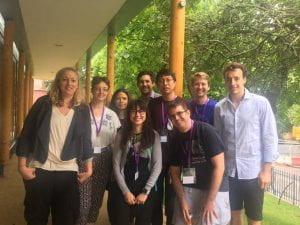
Using AI and machine learning to increase understanding of cardiac muscle proteins – the molecular basis of heart disease – is a potentially daunting challenge. But it was one that Turing Fellow Danielle Paul, from the School of Physiology, Pharmacology & Neuroscience at the University of Bristol, was keen to explore. Danielle took part in the first Turing Network Data Study Group, hosted by the Jean Golding Institute at the University of Bristol – one of The Alan Turing Institute’s 13 partner universities. The event united six Challenge Owners with 50 students, postdocs and senior academics to tackle real-world data science challenges spanning a variety of fields, from spectroscopy and analytical chemistry to text mining and digital humanities.
Building on the popular Data Study Groups (DSGs), held three times a year at Turing HQ in London, this ‘Turing Network’ event was the first of its kind to be hosted by a partner university. It followed the tried-and-tested format of a five-day collaborative hackathon. The Challenge Owners – organisations from industry, government and the third sector – provided real-world data challenges that were tackled by small groups of highly talented researchers. The results were presented on the final day.
Here, Danielle tells us about her experience of the DSG, and the challenge she presented: Applying AI and machine learning to reveal the molecular basis of heart disease.
What was your challenge about?
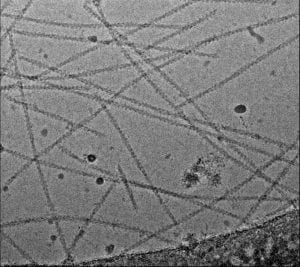
This was an image-processing challenge with potential outcomes that could improve our fundamental understanding of cardiac muscle proteins. The proteins in our images are susceptible to mutations that cause hypertrophic cardiomyopathy, which is a known cause of adult sudden death.
To obtain high-resolution molecular models of these proteins we need to collect hundreds of thousands of images of our protein from noisy data obtained via cryogenic electron microscopy (cryo-EM). It took us six months to manually annotate the small dataset we used in the DSG. It’s a laborious process and it highlights the pressing need for a robust, machine-based approach. If we can automate the protein-identification step, it would overcome a significant bottleneck in our image-processing workflow.
What solutions did the challenge team generate?
The team implemented several deep learning algorithms, known as Convolutional Neural Networks, which were then trained to recognise our proteins in the images. The automated methods that they presented to us performed very well and upon testing, providing as much as 90% accuracy.
What are your hopes for potential applications of the findings from the week?
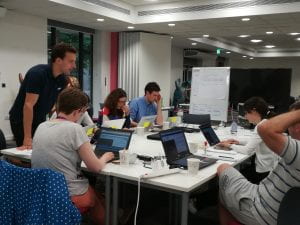
I hope that the various methods that were implemented and trained using our challenge dataset can now be put through their paces on our larger datasets. It will be interesting to see how they perform with slightly different data and imaging conditions. This methodology will be built upon as part of a Turing-funded research project to make a more general software tool to identify proteins in Cryo-EM images.
As a Challenge Owner, what was your favourite part of the Turing Network DSG?
My favourite part was having discussions with the participants. In particular, hearing the ideas and thoughts they had in response to the problems described in our image processing workflow. I appreciate how much effort they put in and their enthusiasm towards addressing the challenge.
Were there any surprises during the event?
That the participants were keen to do more at the end!
Find out more about Data Study Groups, including how you can get involved as a researcher or Challenge Owner
The Alan Turing Institute
The Alan Turing Institute’s goals are to undertake world-class research in data science and artificial intelligence, apply its research to real-world problems, drive economic impact and societal good, lead the training of a new generation of scientists and shape the public conversation around data.