Non-invasive imaging of the eye to predict Alzheimer’s disease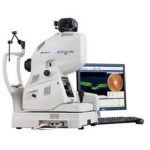
Alzheimer’s Disease (AD) is an increasing global health burden but despite intense research efforts, drug trials have shown little evidence of success. Thankfully, there is now exciting evidence that specialist imaging techniques like optical coherence tomography (OCT) can identify individuals at high risk of developing AD. OCT is a rapid low-cost and non-invasive way to take high-resolution (3-5mm) images of the retina and optic nerves at the back of our eyes and detect early signs of neurodegeneration. It is a technique that is also available in most high street opticians. By using this technique to identify high-risk individuals before they get AD, they have the opportunity to change their lifestyles or enter drug trials at a much earlier stage.
Our aim was to find out how early signs of neurodegeneration in the eye are linked to AD. Using seed corn funding from the Jean Golding Institute, we learnt that measurements of the optic nerves at the back of our eyes can help to determine our future risk of AD. Optic nerve size is associated with eye and brain growth, education, and myopia (short-sightedness). The outcome of our analysis was that people who are more educated and who are more likely to be short-sighted have the lowest risk of AD. Therefore, having to wear glasses is not so bad! Our plan is to run further analysis on other lifestyle and environmental factors that could influence our risk of AD.
If you would like to learn more about this study, please contact denize.atan@bristol.ac.uk
Dr. Denize Atan, Consultant Senior Lecturer, Bristol Medical School (THS)