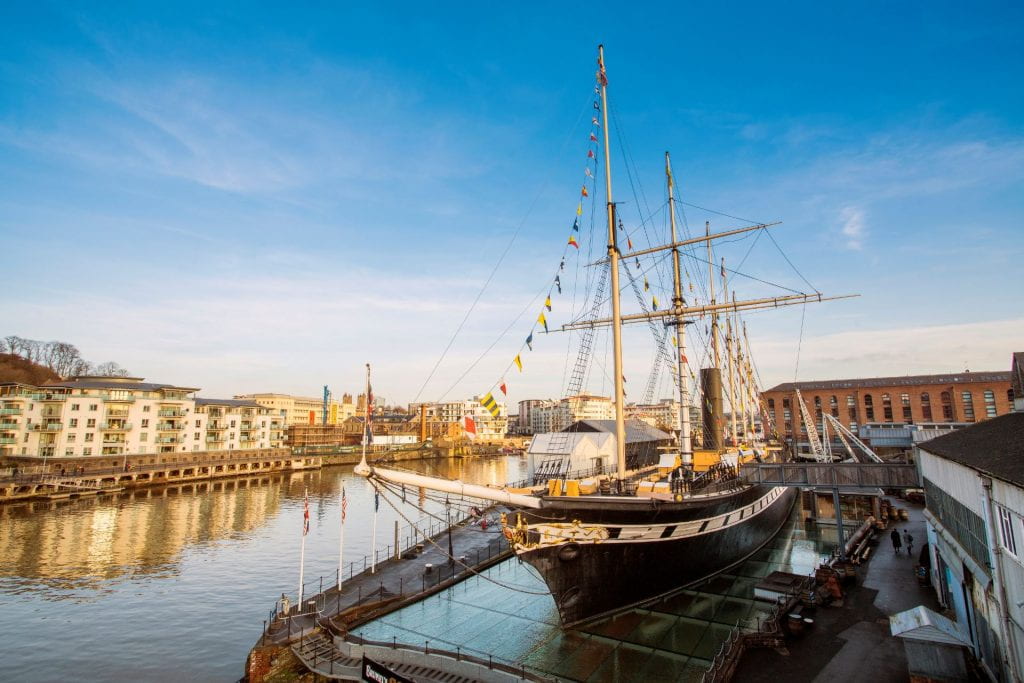
A blog written by James Boyd, Brunel Institute
Isambard Kingdom Brunel
As many Bristolians know, during the 19th century, Isambard Kingdom Brunel was a major force in the development of the city, and the wider region. Working on railways, docks, bridges and revolutionary ships that forged connections across Britain and the world, many of his works are with us today. One of the most significant is the SS Great Britain, which today lies in the heart of Bristol’s old city docks, on the very spot it was built. The first ocean-going vessel in the world to be built of iron, and the first to be moved by a propeller, it is the prototype of the modern ship. Restored as a museum vessel, and visited by hundreds of thousands every year, it is one of the city’s major visitor attractions, and an icon of maritime history.
Brunel Institute
In 2010, the SS Great Britain Trust and the University of Bristol entered into a collaboration to promote and support educational, academic and professional studies in maritime, scientific, industrial and technological history, archaeology and ethnography, through the creation of the Brunel Institute. Situated in the Great Western Dockyard alongside the ship, the Institute is a dedicated facility encompassing archives, reading and teaching space and state of the art conservation suite, which houses the collections and resources of the SS Great Britain Trust, and the University of Bristol’s Brunel Collection, donated by the family in 1950.
Since 2018, James Boyd, resident research fellow at the Institute, has been using its collections, in combination with other major national collections, to piece together the wider network of engineers, investors and patrons involved in the creation of I K Brunel’s three path-breaking steamships. These ships, the Great Western, (1838) Great Britain (1843) and Great Eastern (1859) were each critical to global history. The first of them was the very first ocean-going vessel purpose built to steam non-stop between Britain and North America; the second, as mentioned, transferred ocean ships to modern materials and propulsion; the last was a costly failure for its investors, a hugely oversized vessel for voyaging to Asia Pacific, but it ended up laying the first continual, working telegraph cable connecting Europe and America.
Brunel’s Network
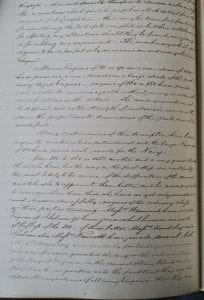
Brunel’s Network is a project that aims to find, record, assess and weight the influence of all the individuals with whom Brunel collaborated in order to deliver these projects. It is an analytical enquiry into communities of innovation, and how they functioned in the past, with Brunel at the epicentre. The analysis initially utilised some basic static network visualizations, built in Gephi, in order to construct a picture from the source material of who provided significant contributions and connections within each project. However, the visualisations and data needed greater dexterity, and also aimed to have a significant public engagement angle, by making interactive, temporal network diagrams available for public exploration.
In a great example of the active collaboration fostered by the Institute, this dynamic element has been generated and continues to be developed by Christopher Woods, Head of Research Software Engineering at Bristol. Christopher has taken the opportunity to create software capable of temporal network analysis that not only comprehends Victorian social, political and professional interactions, but has significant ongoing potential in general temporal analysis of human networks and their development.
With funding support from the Jean Golding Institute, Christopher has also been able to add a third team member, Gareth Jones, so that a public exhibition of Brunel’s Network will be available in the Brunel Institute from the 19th of July 2020 – the 50th anniversary of the day the historic SS Great Britain was towed back to Bristol for restoration. The project team are aiming to have all data, visualisations and analysis prepared for the launch of an interactive app by the close of 2020, before the findings, methods, outcomes (and lessons learned!) are collated and presented to both the digital humanities and data science communities. Hopefully, the project will demonstrate ways in which historical source material and digital methodologies can work in harmony to help both the academic world and wider public comprehend the past, whilst generating present-day software innovations that expand the analytical tools available in Bristol and beyond.
Further progress of this project will be reported in future blog posts.
To keep up to date with this and other projects, news, events, funding and other opportunities please Join the JGI Mailing list.