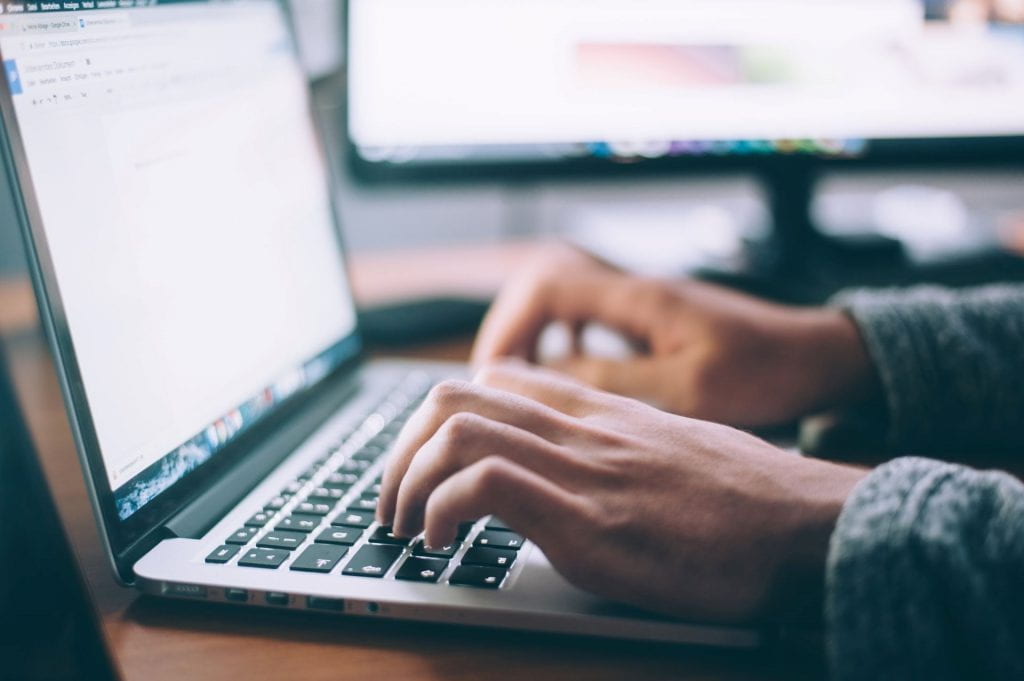
Blog written by Jonty Rougier, Lisa Müller, Soraya Safazadeh, Centre for Thriving Places (the new name for Happy City)
What makes a good spreadsheet layout?
We were recently trying to extract some data from the All tab of the ONS spreadsheet Work Geography Table 7.12 Gender pay gap 2018
This gave us the opportunity to reflect on what makes a good spreadsheet layout, if you want to make your data easily available to others. The key thing to remember is that the data will be extracted by software using simple and standardised rules, either from the spreadsheet itself, or from a CSV file saved from the spreadsheet. Unless you recognise this, much of your well-intentioned structure and ‘cool stuff’ will actively impede extraction. Here are some tips for a good spreadsheet:
Names
Each of your data columns is a ‘variable’, and starts with a name, giving a row of variable names in your spreadsheet. Don’t use long names, especially phrases, because someone is going to have to type these later. Try to use a simple descriptor, avoiding spaces or commas; if you need a space or some other punctuation, use an underscore instead (see below). You can put detailed information about the variable in a separate tab. This detailed information might include a definition, units, and allowable values.
In our example spreadsheet we have
Current column name | Our description | Better column name |
Description | Region names | Region_name |
Code | Region identifiers | Region_ID |
Gender pay gap median | Numerical values | GPG_median |
Gender pay gap mean | Numerical values | GPG_mean |
There is a mild convention in Statistics to use a capital letter to start a variable name, and then small letters for the levels, if they are not numerical. For example, the variable ‘Sex’ might have levels ‘male’, ‘female’, ‘pref_not’, and ‘NA’, where ‘pref_not’ is ‘prefer not to say’, and NA is ‘not available’.
- Use an IDENTICAL name for the same variable if it appears in two or more tabs. It’s amazing how often this is violated: identical means identical, so ‘Region_Name’, ‘region_Name’, and ‘region_name’ are NOT the same as ‘Region_name’.
- There are two different conventions for compound variable names, like ‘Region name’. One is to replace spaces with underscores, to give ‘Region_name’. The other is to remove spaces and use capitals at the start of each word, to give ‘RegionName’, known as camel case. Both are fine, but it is better not to mix them: this can cause some old-skool programmers to become enraged.
Settle on a small set of consistently-used codes for common levels
NA for ‘not available’ is very common; in a spreadsheet, you can expect a blank cell to be read as NA. ‘Prefer not to say’ comes up regularly, so settle on something specific, like ‘pref_not’, to be used for all variables. The same is true for ‘not sure’ (eg ‘not_sure’).
At all costs, avoid coding an exception as an illegal or unlikely value, like 9, 99, 999, 0, -1, -99, -999; we have seen all of these, and others besides (from the same client!). If you want to use more exceptions than just NA in a variable with numerical values, then use NA for all exceptions in the values column, and add a second column with labels for the exceptions.
In our example spreadsheet, if you look hard enough you will see some ‘x’ in the numerical values columns. We initially guessed these mean ‘NA’, but in fact they do not! In the key, ‘x = Estimates are considered unreliable for practical purposes or are unavailable’. But surely ‘unreliable’ and ‘unavailable’ are two different things? Ideally only the second of these would be NA in the GPG_median numerical column. A new GPG_median_exception column would be mostly blank, except for ‘unreliable’ where required to qualify a numerical value.
Generally, we prefer a single column of exceptions, possibly with several levels. In another application the exception codes included ‘unreliable’, ‘digitised’, ‘estimate’, and ‘interpolated’.
Put all meta-data ABOVE the rows which store the data
This is because extraction software will have a ‘skip = n’ argument, to skip the first n rows. So everything which is not data should go up here, to be skipped.
- DO NOT use the columns to the right of your data: the extraction software will not understand, and try to extract them as additional columns.
- DO NOT use the columns underneath your data, for the same reason. Your variables will be contaminated, usually with character values which stop the columns being interpreted by the extraction software as numerical values.
In our example spreadsheet, there is a ‘Key to Quality’ to the right of the columns. Clearly the author of this spreadsheet was trying to be helpful, but this information is already in the Notes tab, and the result is distinctly unhelpful.
In our example spreadsheet we also have three rows of footnotes immediately underneath the data. The correct place for these is in the Notes tab, or above the data.
Do not embed information in the formatting of the cells
This is an unusual one, but our example spreadsheet has done exactly that. Instead of an additional column Quality, the author has decided to give each numerical value cell one of four colours, from white (good quality) to deep red (either unreliable or unavailable). This is useful information but it is totally inaccessible: cell format like colour is not read by extraction software.
Don’t have any blank rows between the row of variable names and the last row of data
This is not crucial because extraction software can be instructed to skip blank rows, but it is better to be safe.
Our example spreadsheet has no blank rows – result!
More information
For more information about Centre for Thriving Places check out their website